The accelerated digital transformation during recent years has created a complex ecosystem for Service Integrators to manage. In this blog, author Biju Krishna Pillai S writes about how SIAM in order to manage these complexities needs the best of the both worlds – a traditional operating model amalgamated with SIAM principles and the flexibility of a digital storyteller by yoking the information capability in a mistake-proof manner.
Introduction
Increased emphasis on IT transformation across organizations has created a complex digital ecosystem for SIAM to manage. SIAM operations are swiftly evolving as digitally-driven practices due to increased adoption rates of:
- Cloud Technologies
- DevOps and Automation
- Pressure for quicker Business to IT alignment
- Fast-paced technology changes
- Realities of the post-pandemic world
Embracing this digital journey comes with being agile and adaptable to overcome the risk of spending too much of time for decision making and organizational change management. A good Service Integrator therefore must be an excellent storyteller by using the information effectively to persuade, relay key messages, and educate on key challenges in an easy-to-digest format across this complex digital ecosystem. How will a Service Integrator perform this role if the underlying information required to create compelling stories is not available with a certain degree of quality? Data, accessibility and quality is therefore undoubtedly the most critical element of a SIAM ecosystem to create agile, data driven decisions ensuring the ability to pivot and improve as required.
Dan Heath, American bestselling author, speaker, and fellow at Duke University one said.
“Data are just summaries of thousands of stories- tell a few of those stories to help make the data meaningful”

This underlines the importance of Service Reporting to aid timely decision-making in the SIAM ecosystem. With many service providers, data and information need to be transferred between parties during service delivery in an ecosystem. If information flow is not supported with the appropriate data, there can be disconnects leading to performance issues and operational chaos. In short, not having the ability to access meaningful, accurate, and timely information for all partners will paralyze the SIAM ecosystem no matter how well the SIAM model has been implemented. Hence the quality of data becomes the paramount catalyst for SIAM’s success.
Deciphering the Data Quality
Data quality is often driven by perceptions or enforcing tactical mistake-proofing using tool configurations in Service Integration process operations scenarios. For example, an Incident record status must be updated as ‘Resolved’ in the service management platform before closing the ticket. Other examples are the use of ‘templates’ to guide data entry during service integration scenarios (like the use of Incident templates or Change models).
However, this author believes such an approach doesn’t give the level of intuitiveness or invasiveness to guarantee data quality. This is because, a binary approach to data quality – whether the data is available or not -is not very realistic due to the multidimensional characteristics of the data with respect to its several dimensions like accuracy, completeness, etc. This paper is an attempt to identify the critical dimensions of data quality to amalgamate a high-level approach for any SIAM implementations to contemplate.

Furthermore, the author strongly evangelizes incorporating relevant sections in SIAM BoKs to provide thought leadership on overlaying a data quality framework and architectural guidelines for SIAM practitioners.
Unlocking the power of SIAM Service Reporting
It is common sense that the SIAM Service Reporting function must be carefully crafted and implemented. A service reporting process framework and technology solution is often a standard offering of most specialist SIAM providers. Is that enough? As service reporting can only slice and dice the data available; there is no guarantee that it will be accurate and represent the actual ground realities. Without a systematic approach to tackling this, over time it will lead to a stagnant ecosystem. Typical symptoms of such ecosystems are:
- Lack of operational transparency leading to misalignment of perception Vs reality
- Loss of business trust that SIAM can deliver or improve service quality
- Lack of consistent and accurate statistics on service performance
As predictive analytics comes of age, organizations are more interested in what might happen rather than what has happened. It is therefore paramount that SIAM Service Reporting should be based on high-quality data which can be consistent, trusted, and verified. The objective of service reporting must be to build competitive advantage by catering real time information at the fingers tips, resulting in proactive intervention before issues happen. To achieve this SIAM must be able to predict whether a service is going to meet the SLAs for the period and if not, initiate corrective measures before it is too late. This enhances organization to realize ‘value’ by adopting a strategy of transforming the historical metrics-based reporting to more predictive and real-time information management.
Another compelling factor to investing in a data quality approach is, that when the service integrator has accountability to govern service providers, the service integrator should also perform management of quality. In an evolving SIAM ecosystem, service providers will be in differing data maturity levels and a systematic data quality approach is required to ensure gradual standardization and uplifting. Although it is highly probable that each individual service provider may have its own data quality measurement capability, this does not represent the integrated view of data across the ecosystem. An overarching Data Quality framework therefore should be established when building the SIAM function. As a ‘rule of the club’, all Service Providers must agree to align their data quality capabilities to this.
The multidimensional perspective of data quality
Data quality can be referred to as the state of qualitative or quantitative pieces of information. There are several definitions of data quality, but data is normally considered high quality if it is fit for the intended use like governance, decision making, and strategy planning. This is not something that can be achieved without performing the below activities.
- Assess the data to understand its current state and distributions
- Check conformity to acceptable data standards or dimensions
- Keeping track of data quality and correcting or preventing deviations
As mentioned above due to the characteristics and the nature in which the data is being used in Service Integration scenarios it is challenging to measure the data quality in binary terms, or in other words; to indicate the data is good or bad. The importance of adopting a multidimensional approach by defining a bare minimum set of quality dimensions should be the pragmatic approach.

For example, can we inspect a data set for its completeness, accuracy, consistency, and fitness (to use)? It is possible to define many other such dimensions based on at what level of invasive data quality measurements are intended. Below provided is the definition of some of these quality dimensions as referred from the public domain:
- Consistency of data is the degree to which data is aligned such as its intended value, range, format, and type as applicable
- Accuracy is the level to which the data is error-free and appropriately represents the characteristics of the process, function, or activity for which the data is intended to use.
- Completeness is the extent to which data is not missing and is of sufficient breadth and depth for the purpose.
- Fitness for use is the extent the data is relevant, appropriate for the intended purpose, and meets specifications.
‘Food for thought’ during practical applications
Although a detailed narration of a SIAM data quality implementation is not in the scope of this paper, the below information can be used as ‘food for thought’. Like any other framework, a SIAM data quality framework also cuts across the layers of People, Process, Product, and Partners. The two major components to achieve these are:
- Digital capacity to aggregate and report data quality
- Data governance through an operating model for the management of data quality
Digital capacity is envisioned to build an aggregate data quality reporting which will have a connected view of data across all service providers. Digital capacity must be considered and enabled to measure the above-mentioned quality dimensions of Consistency, Accuracy, Completeness, and Fitness for use.
The basic building blocks of this start by identify the critical quality fields for each process area. For example – a configurations item (CI) must be attached to every incident record, a root cause analysis must be attached to problem records, etc. Once the critical quality fields are identified the next step is to identify the type of checks to be performed.
For example, to measure completeness, the check can be as simple as measuring the ‘null’ values against a field in the data extract whereas it must contain a value other than ‘null’. Similarly, to measure the consistency of a process the ‘format’ and ‘range’ of the data can be checked. For example, an incident closure field must contain a date and time stamp to be qualified as good data based on ‘format’ and ‘range’ checks. The final step is to tabulate and publish the metrics and facilitate drilling down to the at the granularity to identify causes and devise corrective and /or preventive actions. Below provided is a sample representation of a single incident record overlayed by various checks to measure the quality dimensions.

Famous English writer and philosopher John Ruskin once said “Quality is never an accident. It is always the result of intelligent effort.”
Hence Service Integrator, Client, and Partners must invest collaborative wisdom to develop a technology outline to model the data quality digital capacity. In this digitally-powered era, there are several commercial of the shelf applications available for data aggregation, validation, and presentation with inbuilt analytics and automation capabilities. Below provided is a primitive conceptual schematic of data quality digital capacity to explain the thought process of the author better.
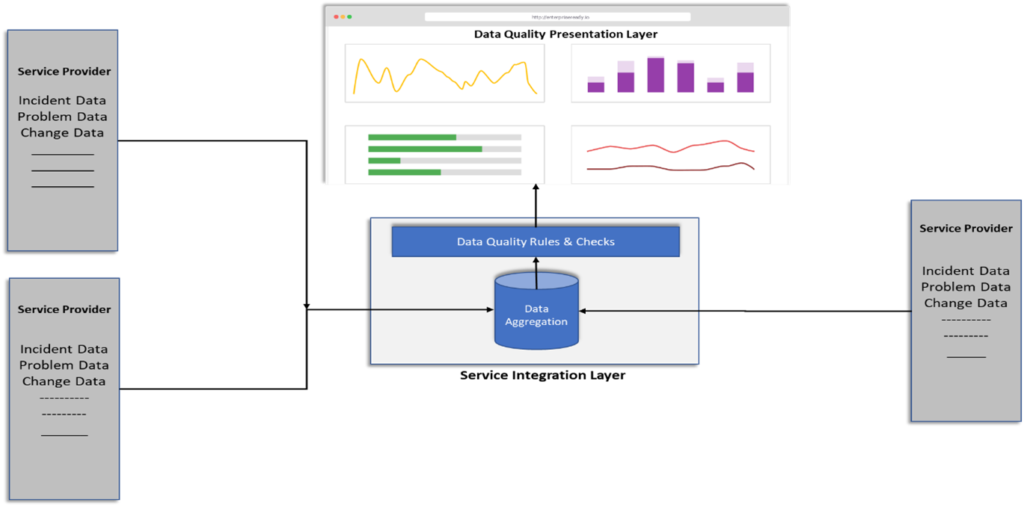
Conceptual schematic of Data Quality digital capacity
Uplifting and sustaining the data quality in the SIAM ecosystem is a continuous process. Data governance should be part of the operational regime of the SIAM ecosystem. A systematic governance structure is required as a method to establish and maintain accountability. As part of this, it will be effective if a target operating model (TOM) with prescriptive roles and responsibilities can be defined
Data Supervisor Group (DSG) – The primary responsibility of DSG is to ensure data defects are identified and remediated in a timely manner. To achieve this DSG should promote data literacy within the ecosystem and encourage the use of the digital capability. SIAM ecosystem will look up to DSG for the guidelines and strategy to drive data quality and sponsorship to use technological capabilities to achieve a quick turnaround
Data Custodians – The primary responsibility is dispensing leadership and direction to resolve any identified data quality defects. Data Custodians should also be responsible for ensuring the integrity of data, allocating resources, and handling escalations and disputes with respect to the data supervision process.
Data Operators – Data Operators are directly responsible for tactical data quality defect identification and triaging. They should collaborate with Data Custodians and provide needed inputs to initiate remedial activities.
At the tactical level Data governance process should consist of the below stages:
- Data quality measures for each process area are defined
- Assess data quality through digital capability using a range of dimensions. The number of dimensions related to each data element will vary based on the nature of the data and the requirements.
- In case of failure of any data quality measures, identify this as a data defect and triage for remediation as per the defined governance structure.
This indicates the need to develop an operational function in line with Service Integration principles and amalgamate with all critical integration activities that the Service Integrator is responsible for.
Conclusion
The accelerated digital transformation during recent years has created a complex ecosystem for Service Integrators to manage. To manage these complexities, SIAM needs the best of both worlds; a traditional operating model amalgamated with SIAM principles and the flexibility of a digital storyteller by yoking the information capability in a mistake-proof manner. Arming SIAM during this ever-spiralling digital transformation with accurate and timely data must be considered as an insurance for success given the massive investment of money and manpower behind every such project.
About the author

Biju Krishna Pillai S has more than 22 years of experience in Information Technology Consulting and is a contributing author and reviewer of SIAM Foundation & Professional BoKs. He is part of Cloud Infrastructure Services (CIS) of Capgemini and specialises in Enterprise Service Management (ESM). Biju is based out of Melbourne, Australia and played pivotal roles in several large SIAM implementations across the world.
He can be contacted on bijupillai2008@yahoo.com or +61 426063540.